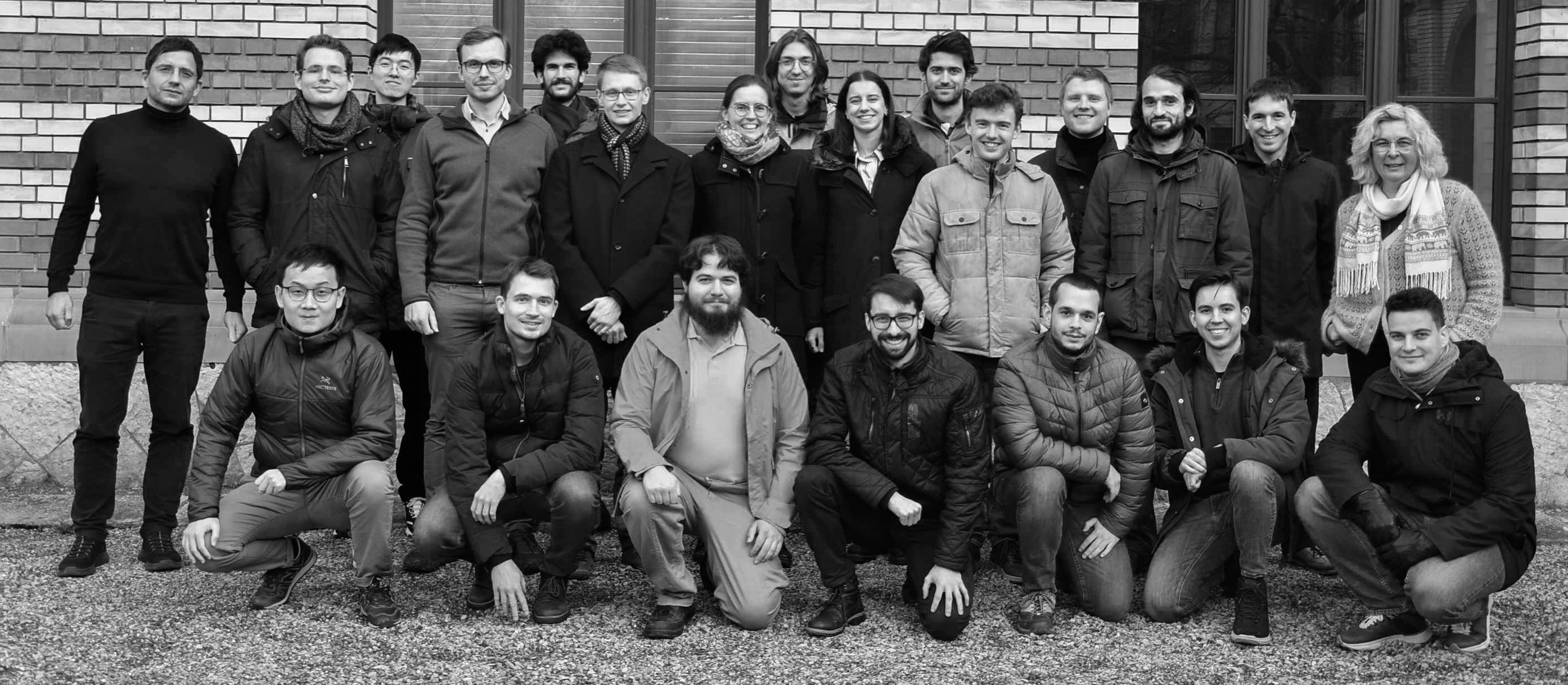
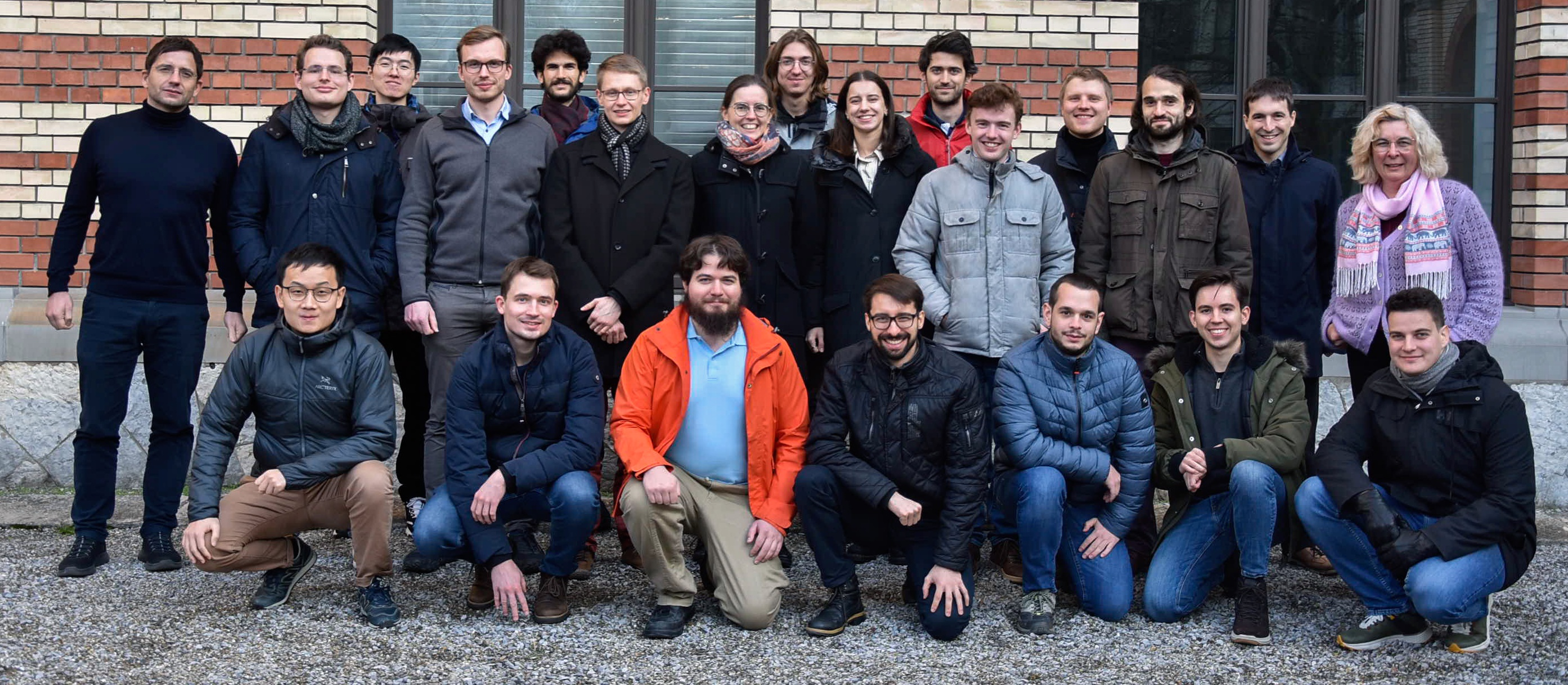
The Secure, Reliable, and Intelligent Systems (SRI) Lab is a research
group in the Department of Computer Science at ETH Zurich.
Our current research focus is on the areas of reliable, secure,
robust and fair machine learning, large language models, probabilistic and quantum
programming, and machine learning for code.
Our work led to six ETH spin-offs:
InvariantLabs (secure AI agents),
NetFabric (AI for systems),
LogicStar (AI code agents),
LatticeFlow (robust ML),
DeepCode (AI for code; acquired by Snyk),
and ChainSecurity (security verification; acquired by PwC).
See our Publications and our Blog to learn more about our latest work, and follow our group on Twitter.
Latest Blog Posts
Latest News
Latest News & Blog Posts
Probing Google DeepMind's SynthID-Text Watermark: We apply the techniques from our recent work to investigate how SynthID-Text, the first large-scale deployment of an LLM watermarking scheme, fares in several adversarial scenarios. We discuss a range of findings, provide novel insights into the properties of this scheme, and outline interesting future research directions.
The Role of Red Teaming in PETs: In February, our team won the Red Teaming category of the U.S. PETs Prize Challenge, securing a prize of 60,000 USD. In this blog post, we will provide a brief overview of the significance of Red Teaming in the field of Privacy Enhancing Technologies (PETs) research in the context of the competition.
LAMP: Extracting Text from Gradients with Language Model Priors: In this work we present an attack on federated learning's privacy specific to the text domain. We show that federated learning in the text domain can expose a lot of user data.
Reliability Guarantees on Private Data: We present Phoenix (CCS '22), the first system for privacy-preserving neural network inference with robustness and fairness guarantees.